June 14, 2023
Esports has evolved into a dynamic and competitive realm. Every move matters. Behind the scenes, player performance data is fueling the drive for success. In this article, we explore how player performance data is essential to the esports landscape.
Get ready to dive into the data-driven world of esports and discover the secrets to unleashing the true potential of players and teams.
The Importance of Player Performance Data
Understanding the Competition
Knowledge is power. Player performance data provides teams and analysts with valuable insights into the competition. By understanding the strengths and weaknesses of their opponents and themselves, teams can devise strategic plans that give them a competitive edge.
Find Strengths and Weaknesses
Player performance data acts as a compass, guiding teams in the right direction. With this compass in hand, teams can navigate their strategies, make informed decisions, and steer toward victory.
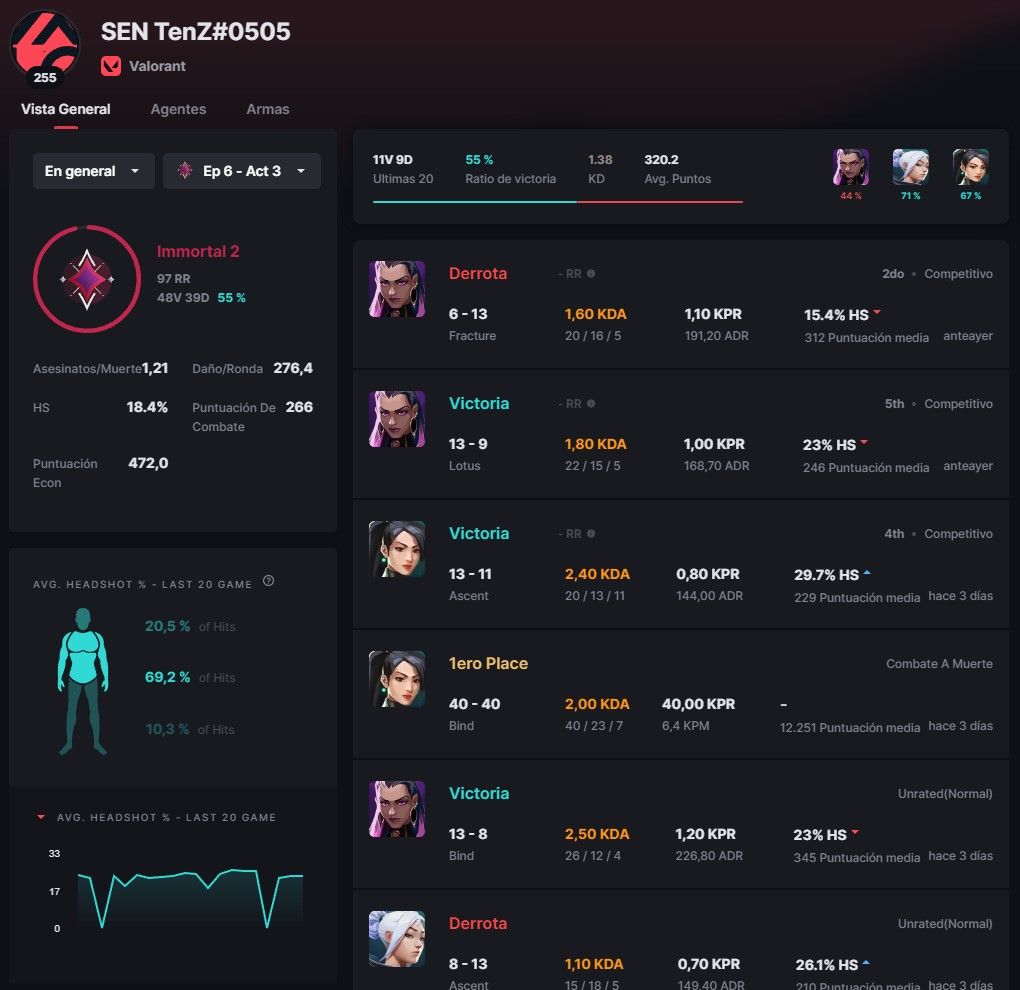
Teams can optimize their gameplay and maximize performance by following a data-driven approach. Multiple metrics depend on the game, such as kill/death ratios, accuracy, objective captures, or average headshot percentage.
Evaluating Individual and Team Performance
Numbers don’t lie. Player performance data allows teams to assess individual and team performance objectively. By tracking key metrics over time, teams can analyze trends, identify consistent performers, and pinpoint areas for improvement. It’s the foundation for developing strategies that bring out the best in players and teams.
Collecting Player Performance Data
In-game Metrics and Statistics
Esports titles often provide a wealth of in-game metrics and statistics. These include kill/death ratios, damage dealt, and more, depending on the game. These metrics are the bread and butter of player performance data. Analyzing them gives teams insights into player effectiveness and allows them to make data-driven decisions.
Data Tracking Tools and Software
To capture more comprehensive player performance data, teams and analysts rely on data-tracking tools and software. These tools tap into the game APIs, telemetry data, and third-party tracking applications, providing real-time and historical data for analysis. Heatmaps and positional data further enhance the understanding of player movement and performance.
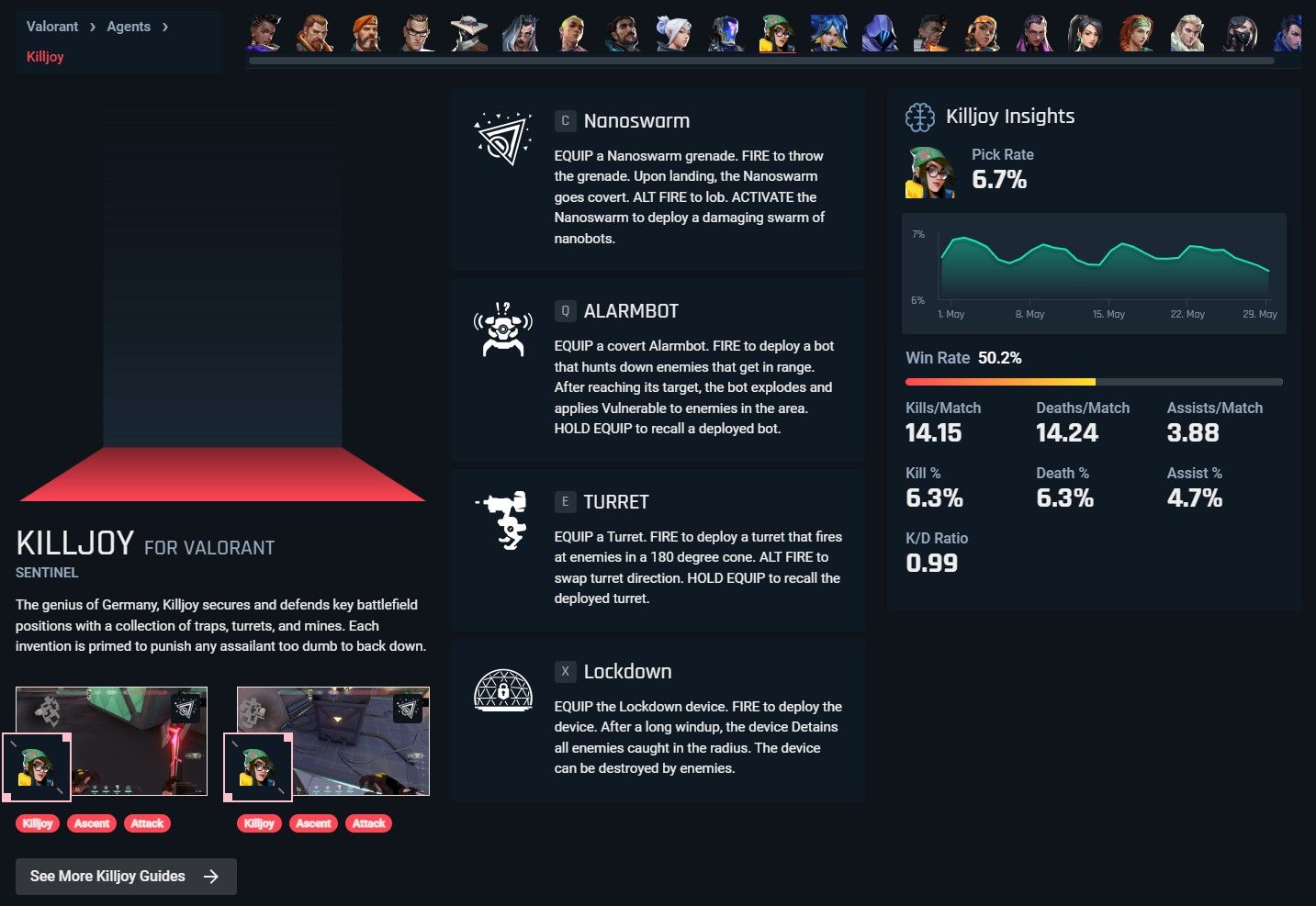
Apps like Blitz or Tracker can even be a good tool for casual players to improve their own individual performance. Apps like Swiftskill even provide heatmaps for Valorant, and Leetify is an in-depth analysis tool for Counter-Strike. I’ve also been researching StatsHelix software, and it seems quite powerful.
Analyzing Player Performance Data
Unveiling Patterns and Trends
Analyzing player performance data uncovers patterns and trends that can shape strategic decisions. Statistical analysis techniques, data visualization tools, and machine learning algorithms enable teams to identify correlations, spot anomalies, and forecast future outcomes. These insights empower teams to make data-informed decisions for gameplay strategies.
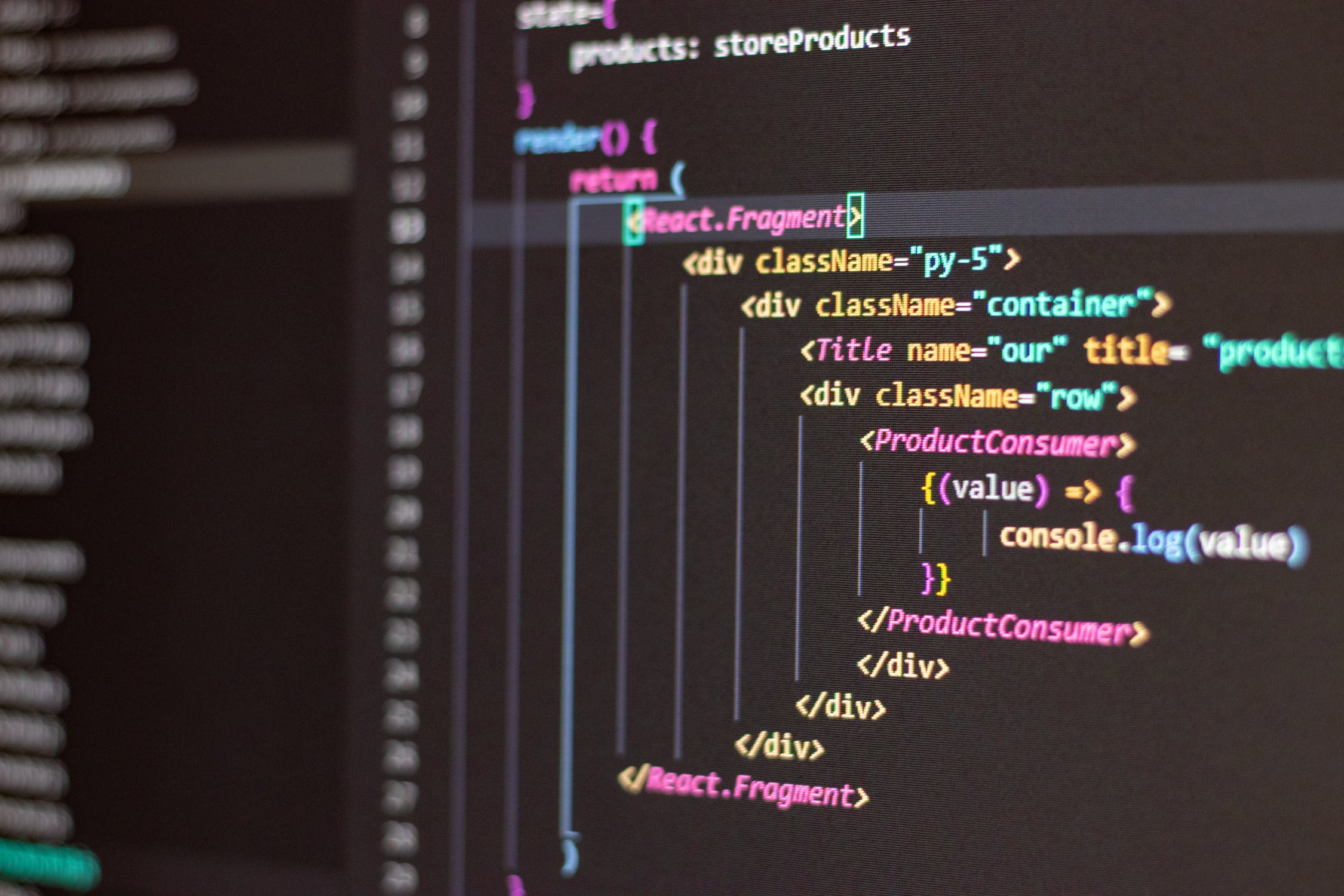
From a computer science student perspective, here are some examples of machine learning algorithms that could be used for unveiling patterns and trends in player performance data:
- Decision Trees: decision trees are versatile algorithms that can identify key factors and create rules for decision-making. In esports, decision trees (https://core.ac.uk/reader/210608830) can be used to analyze player performance data and identify important variables that contribute to success or failure in specific game situations.
- Random Forests: random forests combine multiple decision trees to improve accuracy and robustness. This ensemble learning technique is useful for analyzing complex player performance data and uncovering relationships between different variables.
- Support Vector Machine (SVM): SVM is a supervised learning algorithm that can classify and analyze player performance data based on predefined categories. It can be used to predict player performance or determine playstyles based on historical data.
- Neural Networks: neural networks are deep learning models inspired by the human brain. They can be effective in processing complex player performance data and can uncover intricate patterns and relationships that may not be immediately apparent.
- Clustering Algorithm: clustering algorithms group players based on their performance characteristics, allowing analysts to identify different player archetypes or playstyles within a dataset. This helps in developing targeted strategies and understanding player behavior.
Crafting Winning Strategies
By understanding the strengths and weaknesses of players, teams can optimize their compositions, devise innovative tactics, and exploit opponents’ vulnerabilities. It allows teams to adapt their strategies on the fly and gain a competitive advantage.
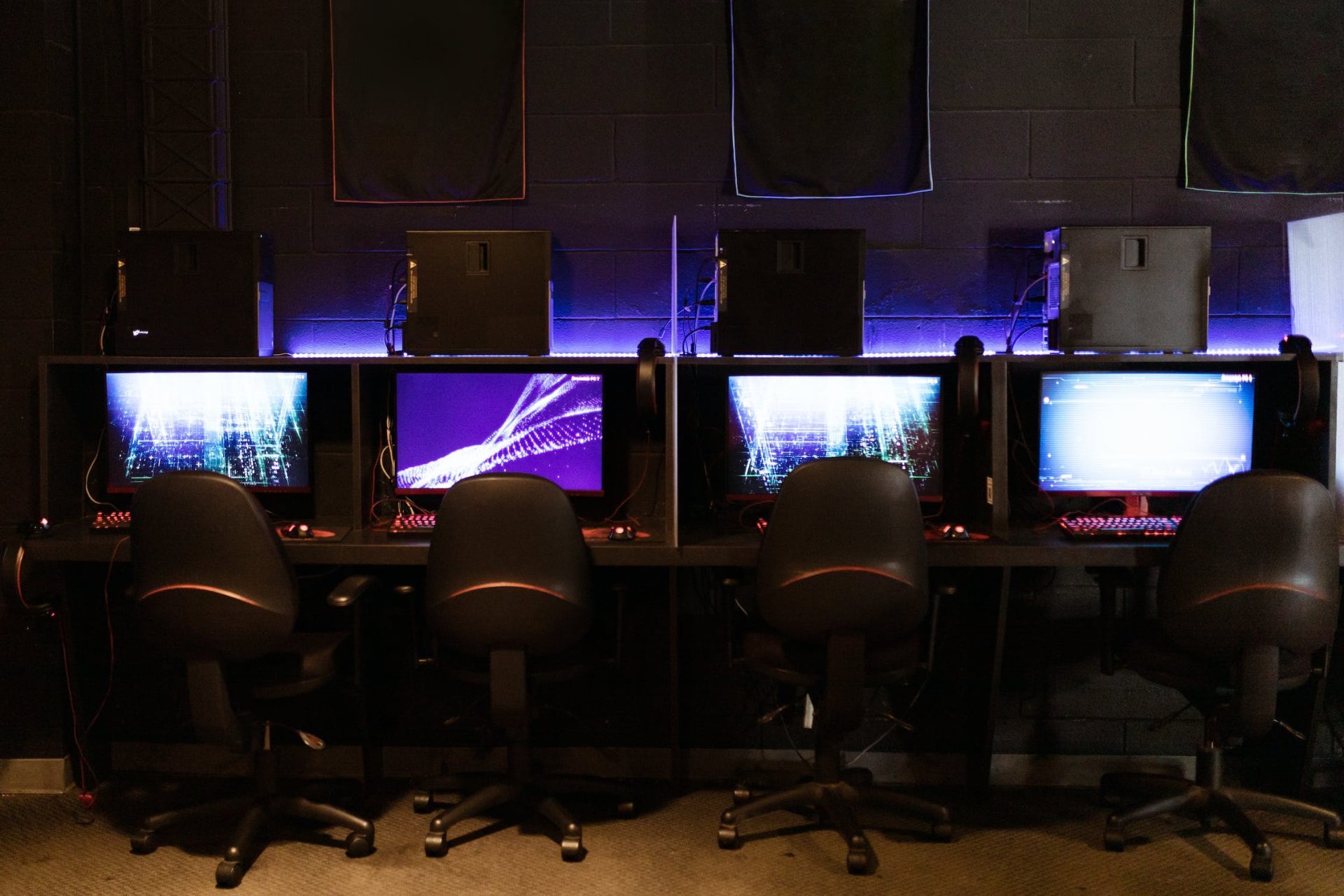
Player performance data facilitates opponent scouting and the development of effective counter-strategies. Analyzing opponents’ performance metrics allows teams to identify playstyles, patterns, and weaknesses to tailor their approaches accordingly. Remember, knowledge is power.
Conclusion
In the dynamic world of competitive gaming, the impact of player performance data cannot be overstated. Its influence has provided teams with invaluable insights for strategic decision-making and player development. By harnessing the power of data-driven analysis, teams can elevate their performance to new levels and maintain a competitive edge in the rapidly evolving scene of esports.